The adoption of AI tools across industries is accelerating. From predictive analytics in finance to chatbots in customer service, businesses are leveraging AI to stay competitive. According to McKinsey & Company’s report titled “The State of AI: How organizations are rewiring to capture value,” 78% of organizations have integrated AI into at least one business function, with a notable increase in generative AI usage from 33% in 2023 to 71% in 2024.
Global AI investment continues to rise, with enterprises allocating substantial budgets to AI-driven solutions. While AI adoption is a strategic move, companies must carefully assess the financial implications to maximize ROI.
In this guide, we’ll break down the complete cost structure of AI tools for enterprises, helping businesses make informed investment decisions.
Why AI Tools Seem Like a No-Brainer but Require a Deeper Look
AI tools often come with enticing promises—automating repetitive tasks, improving accuracy, and generating valuable insights. With user-friendly interfaces and pre-trained models, many businesses assume that adopting AI is a straightforward decision. However, enterprises need to evaluate costs beyond the surface-level pricing to ensure AI aligns with their long-term goals.
Hidden expenses such as data storage, compliance, and vendor lock-in can significantly impact the total cost of ownership. In addition, AI tools require upfront investments, ongoing maintenance, and additional infrastructure, all of which contribute to the overall cost.
To make an informed decision, companies need to consider the following cost factors.
Upfront Costs: Initial Investment in AI Tools
Licensing Fees: Flat, Tiered, or Custom Pricing?
Most AI vendors offer different pricing structures based on enterprise needs. Some charge flat fees, while others provide tiered or custom pricing. Enterprises need to evaluate which model aligns with their usage requirements to avoid overspending.
Hardware Requirements: On-Premise vs. Cloud-Based AI Solutions
AI tools can be cloud-based or require on-premise hardware. While cloud solutions reduce initial capital expenditures, they may lead to higher long-term operational costs due to recurring subscription fees, vendor lock-in, scalability expense, and security add-ons.
On-premise AI solutions demand expensive hardware and maintenance but provide greater control and security.
Customization and Development Expenses
Many AI solutions require customization to fit specific business needs. This customization can include model training, algorithm adjustments, and API integrations—all of which add to the initial costs.Companies may also need to hire developers or consultants to ensure seamless compatibility with existing enterprise software (such as CRM, ERP, or data warehouses).
Hidden Costs: What Companies Overlook
Data Storage and Processing Fees
AI models rely on vast amounts of data, and storing and processing this data comes at a price. Enterprises using cloud-based AI tools must account for storage fees, which can scale rapidly with increasing data usage.
Security and Compliance Costs
Ensuring AI tools comply with regulations such as GDPR, HIPAA, or industry-specific security standards adds another layer of cost. Businesses must invest in cybersecurity measures and legal consultations to avoid compliance risks.
Ongoing Training and Model Updates
AI models need continuous training and updates to remain effective. Enterprises must allocate resources for data labeling, model fine-tuning, and keeping AI systems up to date with evolving business needs.
Vendor Lock-in and Exit Strategy Costs
Many enterprises commit to a particular AI vendor without considering the costs of switching providers in the future. Vendor lock-in can result in high exit costs, making it expensive to transition to a different platform later on.
Pricing Models: How AI Vendors Charge Enterprises
Credit-Based Pricing vs. Seat-Based Pricing: What’s More Cost-Effective?
Some AI vendors charge based on credits (usage-based pricing), while others follow a seat-based model (charging per user). Credit-based pricing works well for fluctuating usage, whereas seat-based pricing is ideal for businesses with a fixed number of users.
Usage-Based Pricing: Paying for What You Use vs. Flat-Rate Plans
Enterprises must decide whether a pay-as-you-go model or a flat-rate subscription is more cost-effective. Pay-as-you-go pricing provides flexibility but can lead to unpredictable costs, while flat-rate plans offer budget consistency.
Freemium vs. Enterprise Tiers: When Do Free Plans Become Expensive?
Many AI tools offer freemium plans with limited features. While these plans seem cost-effective initially, they often require upgrades to enterprise tiers for essential functionalities, leading to unexpected costs.
AI API Costs: Pay-Per-Request vs. Subscription Models
For AI-driven APIs, vendors often charge per request or offer a subscription model. High-frequency usage enterprises should evaluate these costs carefully, as pay-per-request pricing can add up quickly.
Operational Costs: Managing AI in a Corporate Environment
Infrastructure and Cloud Computing Expenses
Managing AI projects often starts with establishing the right infrastructure. Companies must choose between on-premise data centers and cloud-based platforms. Cloud services, while offering scalability, come with recurring costs measured per compute instance, storage, and data transfer. For example, companies might spend anywhere from $0.10 to $1.00 per compute-hour on a cloud platform, depending on the performance tier and usage patterns. Investing in robust infrastructure now can help avoid bottlenecks as AI workloads increase.
In-House vs. Outsourced AI Management
Deciding between an in-house AI team and outsourced solutions is crucial for balancing control with cost efficiency. In-house teams offer deeper customization and seamless integration with existing processes, but require significant upfront investment in recruitment, hardware, and training. Outsourced AI management, by contrast, may lower initial capital expenses and offer faster scalability, though it might involve less direct oversight and potential data security concerns.
Comparison Table: In-House vs. Outsourced AI Management
Source:- Svitla
Maintenance, Updates, and Support Fees
AI systems require ongoing maintenance to ensure they continue to perform optimally. This includes regular updates to algorithms, security patches, and hardware maintenance.
Employee Training and Upskilling Costs
An often-overlooked cost is the continuous investment in employee development. As AI tools evolve, companies must ensure that their workforce remains proficient in the latest techniques. Upskilling programs, workshops, and certifications can cost between $12,000 and $250,000 annually, depending on the number of trainees, depth of training, and the complexity of the AI applications being used.
AI ROI: Are AI Tools Really Worth the Investment?
AI ROI: Cost Savings vs. Revenue Growth: What’s the Real Impact?
AI tools often promise significant cost savings by automating repetitive tasks and optimizing operations. For example, businesses might see a 50% reduction in operational costs alongside a 35% increase in revenue through enhanced customer insights and faster decision-making. However, the precise impact depends on industry, company size, and how well the AI tools are integrated into existing workflows.
Efficiency Gains: How AI Reduces Manual Work
By automating data entry, report generation, and even customer service, AI dramatically reduces the need for manual intervention. For instance, certain AI-driven tools have been shown to reduce manual workloads, allowing employees to focus on higher-value tasks. These efficiency gains not only reduce labor costs but also accelerate decision-making processes.
Industry-Specific ROI Comparisons
Different sectors experience varying levels of ROI from AI investments. For example, the manufacturing industry often realizes significant cost reductions through predictive maintenance and quality control automation, while the retail sector might focus on personalized marketing and inventory management. ROI benchmarks typically range from 20% to 50%, depending on the vertical and the maturity of the AI solution.
Industry ROI Benchmarks
ROI from AI varies significantly across sectors, depending on application areas and solution maturity.
Industry | Typical ROI Range | Key AI Application Areas |
Healthcare | 20-35% | Diagnostic support, patient management |
Technology | 30-45% | Process automation, predictive analytics |
Media & Telecom | 25-40% | Content recommendation, automated customer support |
Financial Services | 30-45% | Fraud detection, risk assessment |
Consumer Goods & Retail | 20-40% | Personalization, inventory management |
Manufacturing | 25-50% | Predictive maintenance, quality control |
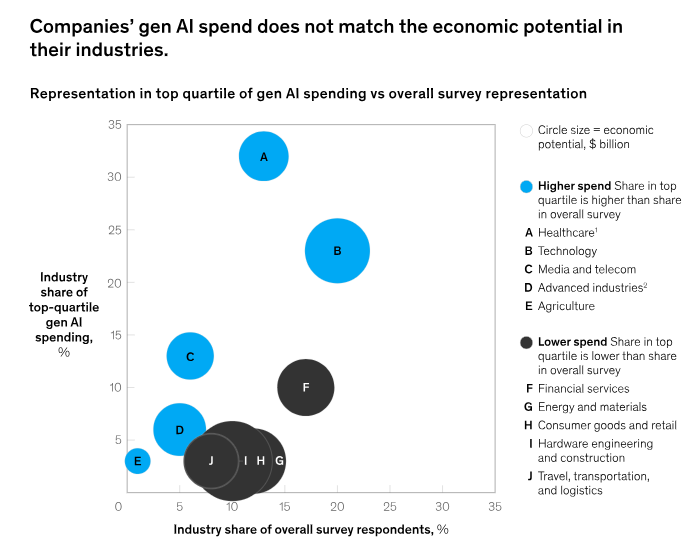
Source: Click Here
Time to Value: How Long Before AI Pays for Itself?
The “time to value” for an AI investment—the period before the benefits outweigh the costs—can vary widely. In Forrester’s Q2 AI Pulse Survey, 2024, 49% of U.S. gen AI decision-makers said their organization expects ROI on AI investments within one to three years, and 44% said within three to five years.
Factors such as integration complexity, employee adaptation, and system robustness all play a critical role in determining this timeline.
Scalability and Long-Term Financial Impact
AI adoption is proving to be a key driver of revenue growth across various business functions. According to recent survey data, 63% of companies report revenue increases from AI adoption in the business units where AI is used.
Companies that are AI high performers—those that effectively leverage AI—are nearly three times more likely than others to see revenue gains exceeding 10%.
Key Revenue-Driving AI Use Cases
AI is most commonly linked to revenue growth in the following business functions:
- Marketing and Sales – AI improves pricing strategies, customer-service analytics, and purchase likelihood predictions, leading to higher conversion rates and increased revenue.
- Product and Service Development – Companies are generating new revenue streams by creating AI-powered products and features that enhance customer experience.
- Supply Chain Management – AI-driven sales and demand forecasting, as well as spend analytics, contribute to more efficient operations and cost savings.
While scaling AI can lead to operational efficiencies, the financial impact is complex. High-performing companies see AI not just as a cost-saving tool but as a revenue-generating asset that enhances sales, innovation, and operational effectiveness.
Scaling Cost Analysis
Source: Click Here
Subscription Inflation: The Rising Costs of AI Tools Over Time
As AI technology becomes more integral to operations, companies may face subscription inflation—where recurring costs gradually rise due to enhanced features, increased usage, or market demand. This inflation can add an extra 5-10% to annual costs, necessitating continuous evaluation of cost-benefit ratios and potential renegotiations with providers.
Subscription Cost Trends
On average, third-party AI tools cost $50 – $10,000 per year (69% of businesses)
Custom AI solutions cost $100 – $5,000 per month (57% of businesses).
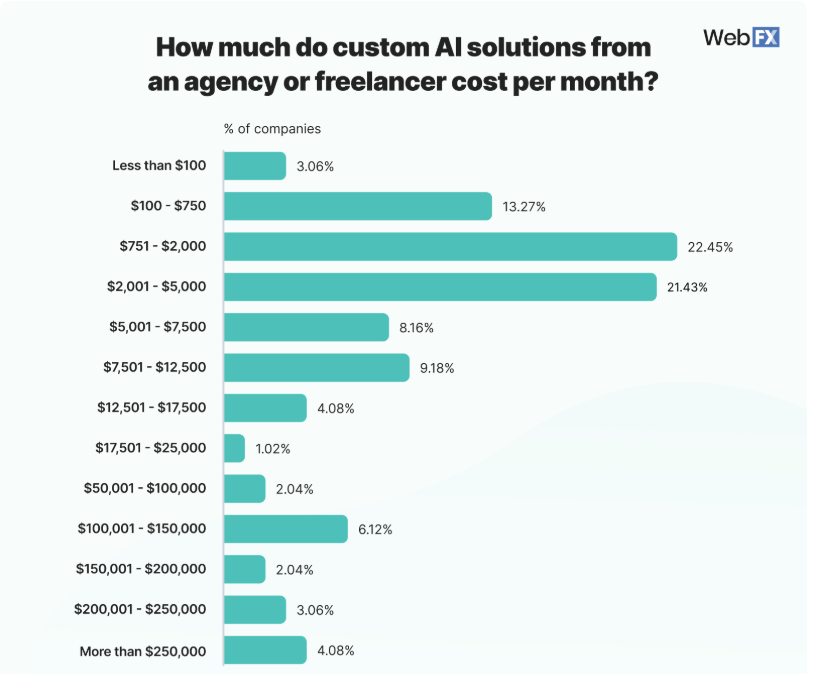
Future-Proofing: Will Today’s AI Investment Stay Relevant?
Future-proofing AI investments is critical given the rapid pace of technological change. Companies need to evaluate whether current platforms can integrate emerging technologies or if the solutions will become obsolete in just a few years. By planning for regular upgrades and modular architecture, businesses can safeguard against rapid depreciation of their AI assets. Strategic investments that allow for scalability and flexibility are more likely to remain relevant.
Case Studies: AI Costs in Real-World Enterprises
Tech Giant vs. SMB: Different AI Cost Structures
AI implementation costs vary significantly between large enterprises and small-to-medium businesses (SMBs). Tech giants, such as Google or Amazon, invest billions in AI research and infrastructure, including proprietary AI models, in-house data centers, and dedicated AI teams. These companies often leverage economies of scale, reducing their per-unit AI costs.
On the other hand, SMBs face constraints in budget and expertise. They typically rely on third-party AI solutions, cloud-based services, or AI-as-a-Service (AIaaS) platforms to stay competitive. While these options lower upfront investment, SMBs may encounter higher operational costs over time due to subscription-based pricing and limited customization.
AI Success Stories: Businesses That Saved Millions
Several businesses have successfully leveraged AI to drive efficiency and cost savings. Here are specific examples of companies that have successfully implemented AI solutions to enhance their operations:
1. Klarna
The Swedish payments firm used AI for customer inquiries, reducing its workforce from 5,000 to 3,800.
2. Amarra
The New Jersey gown distributor uses AI for product descriptions (-60% time) and inventory management (-40% overstocking).
3. 3 Men Movers
The Texas moving company adopted AI for safety, routing, and liability reduction via AI-driven cameras and optimized route planning.
Another notable case is Barclays, a financial institution that implemented AI-powered fraud detection. By analyzing transaction patterns in real time, they were able to identify anomalies and automate case prioritization, allowing for immediate action against potential threats.
Some notable examples:-
1. Barclays
AI-based fraud monitoring detects anomalies in real-time, automating case prioritization for faster threat response.
2. JPMorgan Chase
AI fraud prevention reduced false positives by 20%, improving customer experience and fraud detection.
3. Commonwealth Bank of Australia (CBA)
AI-driven solutions cut call center wait times by 40% and scam losses by 50%.
AI Pitfalls: Companies That Overspent Without ROI
Not all AI investments yield positive returns. Some businesses fall into the trap of overinvesting in AI without a clear strategy. A well-known retail chain spent millions on an AI-driven inventory management system that failed due to poor data integration. The project led to inventory mismanagement and lost sales, forcing the company to revert to manual processes.
Another case involves a healthcare startup that deployed an AI diagnostic tool without proper testing. The tool’s high error rate resulted in misdiagnoses, lawsuits, and reputational damage. These examples highlight the importance of aligning AI investments with well-defined business goals and rigorous testing.
Examples:-
1. Humana
A class-action lawsuit filed in December 2023 alleges that Humana utilized an artificial intelligence (AI) model known as nH Predict to wrongfully deny medically necessary care to elderly patients under their Medicare Advantage plans.
2. Pieces Technologies
Pieces Technologies, a Dallas-based healthcare AI company, faced legal action from the Texas Attorney General’s Office over allegations of false and misleading statements about the accuracy and safety of its AI products.
Decision Framework: Choosing the Right AI Tool for Your Business
Cost-Benefit Analysis: When to Invest in AI
Before investing in AI, businesses must assess the potential return on investment (ROI). Key factors to consider include efficiency gains, cost reductions, revenue growth, and improved customer experiences. A good rule of thumb is to invest in AI when automation can significantly reduce manual workload, enhance decision-making, or drive competitive advantage.
For example, a company processing large volumes of customer inquiries may benefit from AI-powered chatbots, reducing labor costs while maintaining high service levels. However, if AI implementation costs outweigh the expected benefits, delaying investment might be a wiser choice.
Total Cost of Ownership (TCO) vs. Short-Term Savings
Businesses often focus on the immediate costs of AI adoption, such as software licensing and implementation fees, while overlooking the Total Cost of Ownership (TCO). TCO includes long-term expenses such as maintenance, upgrades, training, and infrastructure costs.
For instance, opting for an AIaaS solution might offer lower initial costs but could lead to higher recurring fees over time. Conversely, building an in-house AI system requires a larger upfront investment but provides greater control and lower long-term costs. A balanced approach involves evaluating both short-term affordability and long-term sustainability.
AI Budget Planning: How Much Should You Allocate?
AI budget allocation depends on the industry, company size, and project scope. While enterprises may allocate a sizable chunk of their IT budget to AI initiatives, SMBs must start with pilot projects to test feasibility before scaling up
Key considerations in AI budgeting include:
- Cost of software and AI tools (subscription vs. ownership)
- Infrastructure requirements (cloud computing vs. on-premise solutions)
- Employee training and AI skill development
- Ongoing maintenance and support
Careful planning ensures that AI investments align with business goals without straining financial resources.
Red Flags: Signs You’re Overpaying for AI Tools
To avoid unnecessary expenses, businesses should watch for these red flags:
- Overcomplicated Solutions: Investing in AI with excessive features that don’t align with business needs.
- Hidden Costs: Unexpected fees for integrations, upgrades, or increased usage.
- Lack of Measurable ROI: AI tools that fail to provide clear value in terms of cost savings or efficiency gains.
- Vendor Lock-In: Dependence on a single provider with escalating fees and limited flexibility.
Conclusion
Investing in AI requires balancing cost and value to ensure long-term benefits. While AI enhances efficiency and revenue, hidden costs like integration, security, and training must be considered. Finance leaders and CTOs should evaluate AI investments based on total cost of ownership, scalability, and ROI. Choosing the right pricing model and leveraging cloud-based solutions can optimize spending. A strategic approach to AI adoption ensures maximum business impact while keeping costs manageable and sustainable.
FAQs
What’s the average cost of AI tools for enterprises?
The cost of AI tools for enterprises varies widely based on factors such as functionality, industry, and scale. According to a comprehensive analysis by Walturn, small-scale AI automation projects, like chatbots or rule-based systems, can cost between $10,000 and $50,000. Mid-sized AI projects, such as predictive analytics or natural language processing applications, may range from $100,000 to $500,000. For large-scale, enterprise-grade AI solutions involving complex systems like deep learning or autonomous technologies, costs can exceed $1 million, accounting for extensive research and development, high-performance computing resources, compliance measures, and ongoing maintenance.
Are credit-based AI pricing models better than seat-based ones?
Credit-based models offer flexibility, allowing companies to pay based on usage, making them ideal for businesses with fluctuating AI demands. Seat-based models, on the other hand, provide predictability but may lead to higher costs if AI usage is uneven across employees. The best model depends on the company’s operational needs and AI usage patterns.
What are the best ways to reduce AI implementation costs?
- Leverage pre-trained AI models instead of building from scratch.
- Opt for cloud-based AI services to minimize infrastructure investments.
- Use open-source AI tools where feasible.
- Train employees internally to reduce reliance on costly external consultants.
- Implement AI incrementally, prioritizing high-impact areas first.
How do AI tools compare to traditional software in terms of ROI?
AI tools often provide a higher ROI than traditional software due to their ability to automate complex tasks, enhance decision-making, and improve efficiency. However, traditional software typically has lower upfront costs and predictable maintenance, making it more cost-effective for simpler use cases. AI’s ROI largely depends on the business’s ability to effectively integrate and utilize its capabilities.
What are the hidden costs of AI tools that businesses often overlook?
- Data Preparation and Cleaning: AI models require high-quality data, which involves significant effort and resources.
- Integration Costs: AI solutions often need to be integrated with existing IT infrastructure, which can be costly.
- Talent Acquisition: Skilled AI professionals are in high demand, leading to higher hiring and training costs.
- Compliance and Security: AI implementation must align with industry regulations and cybersecurity standards, adding to overall expenses.
- Maintenance and Continuous Improvement: AI models need regular updates, retraining, and performance monitoring, which incur ongoing costs.