Introduction
Artificial Intelligence (AI) is transforming industries, but its rapid adoption raises serious concerns about data privacy and ethical concerns. AI systems process vast amounts of data, including personal and sensitive information, making them vulnerable to misuse and regulatory violations. Ensuring fairness, transparency, and regulatory compliance is essential for building trust in AI systems. Organizations must address privacy challenges while upholding ethical standards in AI deployment. As AI adoption accelerates, business leaders must take proactive steps to integrate responsible AI adoption into their digital strategies. This involves implementing robust privacy safeguards, mitigating biases, and ensuring compliance with AI privacy laws to protect users and maintain credibility. The ethical implications of AI extend beyond technology and require an interdisciplinary approach involving law, ethics, and data science.
Understanding AI Privacy and Ethical Concerns
What Constitutes AI Privacy?
AI privacy and ethics encompass the protection of personally identifiable information (PII) and preventing misuse of sensitive data. AI systems process enormous amounts of information, ranging from user preferences to biometric data, making them susceptible to breaches and exploitation.
Key privacy risks include:
- Unauthorized data collection: AI-driven applications may collect data without users’ knowledge or explicit consent.
- Lack of user consent: Many AI systems operate with ambiguous consent models, leaving users unaware of how their data is being used.
- Data breaches and cyber threats: AI databases are lucrative targets for hackers, posing risks of identity theft and financial fraud.
Ensuring AI privacy involves embedding security measures at every stage of AI development, from data collection to model deployment. Organizations must integrate privacy-by-design principles and establish stringent access controls to safeguard sensitive information.
The Ethical Dimensions of AI
Ethical AI extends beyond legal compliance and involves making morally sound decisions in AI deployment. Ethical considerations influence AI applications in healthcare, finance, hiring, and law enforcement, where biased algorithms can have severe consequences.
Challenges include:
- Bias in AI decision-making: AI models trained on biased datasets can reinforce discrimination, leading to unfair outcomes in employment, lending, and judicial processes.
- Transparency in algorithmic processes: Many AI models operate as “black boxes,” making it difficult to understand how decisions are made.
- Accountability for AI-driven outcomes: When AI makes mistakes, determining responsibility—whether it lies with the developer, data provider, or business using AI—becomes a complex issue.
Addressing these challenges requires multi-stakeholder collaboration, where businesses, regulators, and academia work together to develop ethical AI frameworks and governance models.
Protection of Personally Identifiable Information (PII) in AI Workflows
Defining PII in AI and Why It’s Critical
PII refers to any data that can identify an individual, such as names, addresses, Social Security numbers, and biometric data. AI systems analyze PII to enhance user experiences, but improper handling can lead to data leaks and regulatory penalties.
Risks associated with AI handling PII include:
- Unintentional data leaks: AI models may inadvertently expose sensitive information if trained on poorly anonymized datasets.
- Unauthorized sharing of user data: Some AI applications share collected data with third parties without clear user consent, raising ethical and legal concerns.
Protecting PII requires organizations to implement stringent policies that dictate how personal data is collected, stored, processed, and deleted after its intended use.
Methods to Protect PII in AI Workflows
To ensure AI data security, organizations can:
- Use data anonymization and pseudonymization techniques: These methods remove or mask identifiable information while preserving the dataset’s analytical utility.
- Implement encryption for AI training datasets: Encrypted data ensures that even if unauthorized access occurs, the data remains unreadable.
- Apply differential privacy to prevent individual identification: This approach introduces statistical noise to datasets, making it nearly impossible to trace data back to a specific individual.
By integrating these security practices, businesses can build AI systems that prioritize user privacy and align with global data protection laws.
Ethical Data Collection and Consent in AI Training
The Importance of Ethical Data Sourcing
Ethical data collection practices ensure compliance with AI privacy laws such as:
- General Data Protection Regulation (GDPR) – Applicable in the European Union (EU) and European Economic Area (EEA), regulating data privacy and protection.
- California Consumer Privacy Act (CCPA) – Applicable in California, United States, giving consumers rights over their personal data.
AI models require vast datasets for training, but not all data is collected ethically.
Best practices include:
- Respecting data ownership and the right to be forgotten: Users should have control over their data and the ability to request its deletion from AI databases, as mandated by GDPR.
- Using AI governance frameworks to regulate data sourcing: AI developers should establish clear guidelines for sourcing data ethically, ensuring diverse representation to minimize bias and promote fairness.
Informed Consent in AI Systems
For ethical AI adoption, companies must:
- Clearly define valid consent: Users should be fully informed about how their data will be used before granting access.
- Use transparent AI data collection policies: Businesses should provide clear, easy-to-understand privacy policies that outline their AI data usage practices.
Failure to secure informed consent can result in legal actions and loss of consumer trust.
Algorithmic Bias in AI
Racial Bias in Hiring AI Tools
In 2018, Amazon scrapped its AI-powered hiring tool after discovering it was biased against women. The system, trained on resumes submitted over a decade, favored male candidates because the majority of historical hires were men. Resumes containing words like “women’s” (e.g., “women’s chess club”) were penalized, leading to gender discrimination (source).
Unfair Lending Decisions in Financial AI
2021 study by the U.S. Consumer Financial Protection Bureau found that some AI-driven credit scoring models disproportionately denied mortgage loans to Black and Hispanic applicants, even when they had similar financial backgrounds to white applicants. This bias stemmed from historical lending patterns embedded in training data (source).
Bias in AI-Based Criminal Sentencing
The COMPAS risk assessment tool, used in U.S. courts to predict recidivism, was found to incorrectly classify Black defendants as high risk nearly twice as often as white defendants. This bias in risk assessment led to harsher sentencing recommendations for Black individuals.
Mitigating AI Bias
To reduce algorithmic bias, organizations must:
- Use diverse and representative datasets during AI training.
- Implement fairness audits and bias-detection tools to identify and correct disparities.
- Follow ethical AI governance frameworks, such as the EU AI Act and NIST AI Risk Management Framework in the U.S.
To ensure fair AI models, companies have implemented corrective measures:
- Fairness-Aware Machine Learning – IBM developed the AI Fairness 360 (AIF360) toolkit, an open-source library that detects and mitigates bias in AI models by applying fairness metrics and corrections (IBM).
- Bias Audits and Regular Testing – The Berkeley Haas Center for Equity, Gender, and Leadership created a playbook guiding companies on detecting and recalibrating AI biases to ensure equitable outcomes (Berkeley Haas).
- Inclusive Data Collection – Companies like Renude and Haut.AI are refining AI-driven skin analysis by expanding datasets to represent diverse skin tones, improving personalization in the beauty industry.
By addressing bias in AI, organizations can create fairer and more reliable AI-driven systems.
Navigating Global Data Privacy Regulations
Businesses handling cross-border AI data must follow key principles like lawfulness, purpose limitation, data minimization, security, and individual rights protection.
Several regulations dictate AI data handling, including:
- GDPR (General Data Protection Regulation) in Europe: Establishes strict rules on how organizations handle personal data. Based on principles of lawfulness, fairness, and transparency, the GDPR enables individuals to access, rectify, erase, port, and restrict processing of their data.
- CCPA (California Consumer Privacy Act) in the U.S.: Grants consumers control over their personal data, including the right to know, delete, and opt-out of the sale or sharing of their data.
- LGPD (Brazil’s Lei Geral de Proteção de Dados): Modeled after GDPR, it grants similar rights to Brazilian citizens.
- CPPA (Canada’s Consumer Privacy Protection Act): Proposes enhanced privacy rights and data protection measures, requiring organizations to be more transparent about how they collect, use, and disclose personal information, including the use of algorithms and artificial intelligence systems.
- PIPL (China’s Personal Information Protection Law): Establishes comprehensive rules to protect the personal information of Chinese citizens, regardless of where it is processed, and applies to organizations and individuals processing such data.
- APEC CBPR (Asia-Pacific): A voluntary, accountability-based program designed to facilitate the safe and compliant flow of personal information across borders within the Asia-Pacific Economic Cooperation (APEC) region.
Compliance Best Practices
- Conduct Data Transfer Impact Assessments (DTIAs)
- Use approved transfer mechanisms such as Standard Contractual Clauses (SCCs), Binding Corporate Rules (BCRs), and Cross-Border Privacy Rules (CBPRs).
- Apply privacy-enhancing technologies like encryption and anonymization
- Maintain records of AI data flows and update compliance programs
How Qolaba Enables Ethical and Compliant AI Usage
Qolaba ensures that personally identifiable information (PII) is never exposed unnecessarily. We use masking, anonymization, and secure handling protocols across all workflows.
SSO Authentication with Leading Identity Providers
We support secure Single Sign-On (SSO) with Google, Azure AD, and GitHub, ensuring frictionless and safe access for enterprise teams.
Enterprise-Grade Data Encryption
All data in Qolaba is encrypted in transit and at rest, using industry-standard AES-256 and TLS protocols.
Role-Based Access Controls (RBAC) & Audit Trails
Admins can configure granular access for team members. All activity is logged with full audit trails to ensure transparency and accountability.
Secure API Integrations & Containerized Deployments
All integrations follow strict API security standards and our deployment model uses containerization and sandboxing to isolate environments.
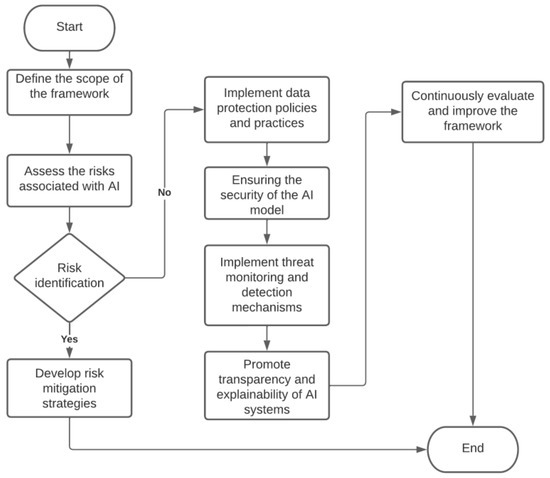
Source: Toward a Comprehensive Framework for Ensuring Security and Privacy in Artificial Intelligence
Conclusion
As AI continues to advance, prioritizing privacy, ethics, and regulatory compliance is essential to maintaining public trust. Organizations must adopt robust data protection measures, mitigate algorithmic bias, and ensure transparency in AI-driven decision-making.
To foster ethical AI, businesses should implement governance structures, conduct regular audits, and engage in proactive risk assessment. Privacy-enhancing technologies and inclusive AI frameworks can help mitigate risks while maximizing AI’s benefits.
A collaborative approach involving policymakers, industry leaders, and technologists is crucial for shaping responsible AI. By promoting interdisciplinary research and global cooperation, we can develop AI systems that align with societal values and human rights. The future of AI should balance innovation with responsibility, ensuring fair, transparent, and ethical implementation that serves diverse populations while upholding individual freedoms.
FAQs on AI Privacy and Ethics
1. Why is AI privacy important?
AI privacy protects sensitive user data from misuse, unauthorized access, and breaches, ensuring compliance with legal regulations and fostering trust.
2. How can organizations ensure AI ethical compliance?
Organizations can ensure ethical AI compliance by integrating privacy-by-design principles, conducting bias audits, implementing transparency measures, and following global AI regulatory frameworks.
3. What are common AI ethical concerns?
Significant AI ethical concerns include bias in AI models, lack of transparency, data privacy risks, and accountability for AI-driven decisions.
4. How does AI bias impact decision-making?
AI bias can lead to unfair and discriminatory outcomes in hiring, lending, and law enforcement due to biased training datasets reinforcing societal inequalities.
5. What are the best practices for protecting AI user data?
Best practices include encryption, anonymization, differential privacy, informed consent policies, and strict access controls to safeguard AI user data.
By addressing these FAQs, organizations can better understand AI privacy and ethics while fostering responsible AI adoption